The Hallucination Paradox: Trust, Verification, and AI's Intuitive Dilemma
What we politely call 'hallucinations' in AI are simply 'lies' in humans. Both stem from overconfident intuition without verification.
Claiming to know something that you cannot or do not know has a specific name in human discourse. We call it lying. Yet when an AI system makes unfounded claims with similar confidence, we've coined the gentler term "hallucination"—a linguistic courtesy we rarely extend to our fellow humans.
This asymmetry reveals something profound about both artificial and natural intelligence. LLMs in their raw completion form represent pure intuition—pattern-matching at impressive scale but without the deliberative verification mechanisms that separate knowledge from mere pattern recognition. Their confident incorrectness mirrors a distinctly human failing.
When Reagan adopted the Russian proverb "доверяй, но проверяй" (trust, but verify) during nuclear disarmament talks, he wasn't just offering diplomatic wisdom. He was articulating a fundamental principle that addresses a shared vulnerability in both silicon and carbon cognition: the dangerous gap between intuitive certainty and verified truth.
The Confidence-Competence Illusion
Modern language models often generate text with unwavering confidence regardless of accuracy. Ask a simple factual question about an obscure historical figure, and the model produces a detailed biography—complete with specific dates, accomplishments, and personal details—whether that person existed or not.
This mirrors what psychologists call the Dunning-Kruger effect in humans. Those with the least knowledge often express the most certainty, while genuine experts acknowledge the boundaries of their understanding. The parallels run deeper than surface similarity.
Both phenomena stem from the same architectural limitation: System 1 thinking (to use Kahneman's terminology) operates without the metacognitive awareness necessary to recognize its own limitations. The spontaneous, intuitive completion of patterns—whether neural or digital—carries no inherent mechanism for self-assessment.
The distinction lies not in the tendency to generate plausible falsehoods, but in our expectations. We've grown accustomed to human error through millennia of shared existence, while the confident pronouncements of AI systems still carry the sheen of technological infallibility despite being constructed from fundamentally similar pattern-matching architectures.
Incentivized Ignorance in Human Knowledge Systems
"It is difficult to get a person to understand something when their salary depends on not understanding it," Upton Sinclair observed—not Lewis as commonly misattributed, an ironic demonstration of the very fallibility under discussion.
Our human knowledge ecosystem creates perverse incentives against accuracy. Medical advice follows funding sources. News optimizes for engagement over truth. Political discourse rewards tribal certainty over nuanced accuracy. Even academic publication incentivizes positive results over failed hypotheses, creating significant gaps in our collective knowledge.
Historical examples abound. Physicians once recommended cigarette brands. Fossil fuel companies buried climate research contradicting their business models. Pharmaceutical companies selectively publish favorable studies while shelving negative results. These aren't mere errors—they represent systematic distortions in our information landscape driven by economic and social incentives.
LLMs trained on this corpus inherit these distortions, reflecting not just human knowledge but human knowledge systems, complete with their endemic biases toward convenient, profitable, or comforting falsehoods. The model doesn't "know" which parts of its training data represent scientific consensus versus corporate propaganda—it simply identifies and reproduces patterns without access to their epistemological foundations.
The hallucination problem thus represents not a failure of technology but a faithful reproduction of a distinctly human problem: we often value plausibility over accuracy, especially when the former better serves our individual or collective interests.
Verification: The Ancient Solution to a Modern Problem
The techniques emerging to address AI hallucinations bear striking resemblance to those civilization has been attempting to instill in humans for millennia:
Internal Dialogue – The Socratic method, dating back to ancient Greece, established questioning as the foundation of knowledge. Modern chain-of-thought prompting asks LLMs to "think step by step" before answering—essentially requesting the same deliberative process Socrates demanded from his students.
External Verification – The scientific method relies on empirical evidence to validate hypotheses. Retrieval Augmented Generation (RAG) similarly grounds model outputs in verified external sources, constraining imaginative completion with factual boundaries.
Metacognitive Awareness – Epistemic humility—acknowledging the limits of one's knowledge—represents a sophisticated human cognitive achievement. We now prompt models to express uncertainty when information is insufficient, teaching silicon the same metacognitive skill we value in carbon minds.
Collaborative Knowledge Building – Peer review in science creates collective validation beyond individual certainty. AI systems increasingly leverage multiple knowledge sources and reasoning paths, mimicking the distributed verification we use to establish scientific consensus.
These parallels suggest that addressing hallucinations isn't a novel technical challenge but the latest iteration of humanity's oldest epistemological project: distinguishing reliable knowledge from plausible fiction.
The Verification Asymmetry
A curious phenomenon emerges when comparing verification practices across natural and artificial intelligence: the standards we're establishing for AI often exceed those we apply to ourselves.
Corporate executives make decisive pronouncements about market trends with far less statistical rigor than we demand from machine learning models. Politicians assert causal relationships between policies and outcomes with confidence that would embarrass a cautious data scientist. News headlines draw sweeping conclusions from limited samples that would never pass algorithmic fairness evaluations.
This asymmetry creates a verification paradox: we're developing rigorous frameworks to mitigate AI hallucinations while simultaneously consuming and producing human communications that would fail those same verification standards. We worry about AI systems confidently stating falsehoods while elected officials and media personalities do precisely the same thing without similar scrutiny.
Perhaps the development of verification mechanisms for AI offers an opportunity to reconsider our tolerance for unverified human certainty. If we can design systems that acknowledge uncertainty, cite sources, and express appropriate epistemic humility, why accept less from human experts and authorities?
Deliberation as the Shared Path Forward
The emerging consensus on addressing AI hallucinations points toward deliberative thinking—techniques that augment intuitive pattern matching with structured verification procedures.
For machine systems, this includes: - RAG systems that ground completions in verified knowledge sources - Multi-step reasoning chains that decompose complex problems - Self-critique approaches where models evaluate their own outputs - Multiple perspective generation to identify inconsistencies
For human systems, we have parallel approaches: - Science and empirical methods for grounding claims in evidence - Critical thinking frameworks that decompose complex arguments - Reflective practices that encourage self-examination of beliefs - Diversity of viewpoint as a method for identifying blind spots
The core principle uniting these approaches is straightforward: intuition proposes, deliberation disposes. Pattern recognition generates plausible answers that deliberative processes subsequently verify, refine, or reject.
The history of human knowledge represents a millennia-long project to develop such deliberative techniques, from Aristotelian logic to Bayesian statistics. The hallucination challenges of AI systems merely highlight the continued importance of this project in an era of accelerated information production.
Trust, Verification, and the Future of Knowledge
As AI systems become increasingly integrated into knowledge production and dissemination, distinguishing between verified and unverified information grows more crucial. The technical solutions emerging for AI hallucinations may ultimately transform human knowledge systems as well.
Imagine citation standards as rigorous for human communications as for AI outputs. Envision political claims automatically cross-referenced against primary data sources. Consider news headlines with confidence intervals rather than unqualified assertions.
The techniques we're developing to make AI systems more truthful might ultimately make human discourse more truthful as well. By building external verification into our information infrastructure, we could address the shared vulnerability of overconfident intuition across both natural and artificial intelligence.
After all, the most transformative technology isn't one that changes what we can do, but one that changes how we think. The verification systems we're building to constrain AI hallucinations might ultimately transform human epistemology itself—not by making machines more like humans, but by making humans more like the idealized, deliberative thinkers we've always aspired to be.
Perhaps the ultimate contribution of AI won't be superhuman intelligence, but rather tools that help us overcome our very human tendency to confuse confidence with competence, assertion with accuracy, and plausibility with truth.
The hallucination problem thus offers a profound opportunity: by teaching machines to verify their intuitions, we might finally learn to better verify our own.
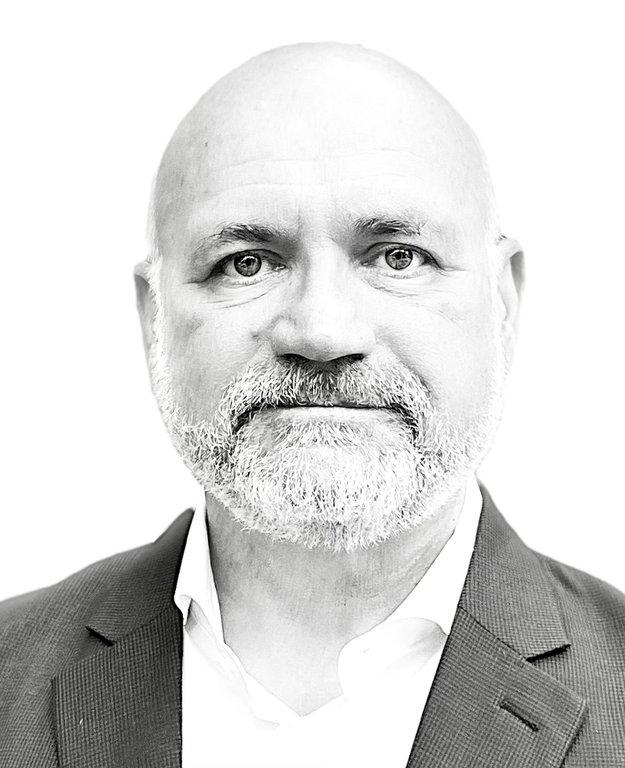
Geordie
Known simply as Geordie (or George, depending on when your paths crossed)—a mononym meaning "man of the earth"—he brings three decades of experience implementing enterprise knowledge systems for organizations from Coca-Cola to the United Nations. His expertise in semantic search and machine learning has evolved alongside computing itself, from command-line interfaces to conversational AI. As founder of Applied Relevance, he helps organizations navigate the increasingly blurred boundary between human and machine cognition, writing to clarify his own thinking and, perhaps, yours as well.
No comments yet. Login to start a new discussion Start a new discussion