Epinomy - Beyond Language Models: Tool-Enabled AI vs. Digital Debris
Organizations struggle with digital debris (ROT data) that wastes resources and creates liability. Modern AI systems with tool access through protocols like MCP provide a solution by enabling governan
Organizations pay approximately five times more than necessary for data storage. Not because hardware prices remain high, but because most enterprises have become digital hoarders—accumulating vast quantities of Redundant, Obsolete, and Trivial information (ROT data) across disparate systems. Research consistently shows that 80-85% of organizational content delivers zero business value yet consumes resources, creates liability, and undermines efficiency.
The persistence of this problem across decades points to a fundamental truth: the tools available for addressing digital debris never matched the scale of the challenge—until now.
Recent excitement around large language models has created a mistaken impression that AI alone can solve the ROT data crisis. This perspective misses a crucial insight: like humans, AI systems achieve their greatest impact not through raw intelligence but through tool use. Just as a human accountant leverages spreadsheets to manage complex financial data, AI systems need specialized tools to effectively govern information at enterprise scale.
The emergence of tool-enabled, agentic AI systems transforms information governance from an insurmountable challenge into a tractable problem. These systems don't merely understand content—they interact with existing enterprise applications, orchestrate complex workflows, and execute remedial actions autonomously.
The Crucial Distinction: LLMs vs. Tool-Enabled AI
Language Models: Cerebral Cortices in Jars
Large language models demonstrate remarkable abilities to understand and generate text, classify content, and reason about information. However, language models operating in isolation resemble cerebral cortices disconnected from bodies—capable of impressive thought but unable to effectively manipulate their environment.
This limitation manifests clearly in information governance scenarios. A standalone LLM might correctly identify redundant documents but cannot access repository APIs to consolidate them. It might recognize outdated policies but lacks mechanisms to update references across systems. It might detect trivial content but cannot implement retention actions.
In essence, LLMs without tool access can diagnose problems but remain powerless to solve them—an insufficient approach for organizations drowning in digital debris.
Tool-Enabled Systems: From Intelligence to Agency
The transformative leap occurs when AI systems gain the ability to use tools—to extend their capabilities beyond understanding into action. This tool use mirrors a fundamental aspect of human intelligence that separates us from other cognitive beings: our ability to create and manipulate external technologies that augment our native capabilities.
Modern agentic AI systems achieve this through structured protocols that enable API-based interactions with enterprise systems. These systems can:
- Access information across disparate repositories through standardized connectors
- Analyze content using specialized tools beyond their native capabilities
- Execute actions through existing application interfaces
- Document processes in auditable, transparent formats
This capability turns theoretical governance into practical action—the difference between identifying a warehouse full of unnecessary documents and actually cleaning it out.
Model Context Protocol: The Missing Link
The recent development of the Model Context Protocol (MCP) represents a watershed moment for information governance. This standardized approach enables AI systems to interact with existing enterprise tools—including document management systems, content repositories, classification engines, and workflow platforms—through a common interface.
MCP solves a critical challenge: how to connect AI systems to the vast ecosystem of specialized information management tools that organizations have already deployed. Rather than requiring wholesale replacement of existing infrastructure, MCP allows AI systems to leverage these investments by providing a universal connector layer.
This approach mirrors how humans interact with specialized software. An information governance professional might use document management systems, classification tools, search platforms, and workflow engines to manage content effectively. MCP enables AI systems to use these same tools, but with greater consistency and at vastly larger scale.
The protocol's standardized approach creates a foundation for composable workflows where multiple specialized tools can be orchestrated into comprehensive governance processes. A single agentic system might leverage document analysis tools to identify ROT candidates, retention calculation engines to determine appropriate policies, legal hold systems to verify disposition eligibility, and repository APIs to execute consolidation actions.
The Tool-Enabled Transformation of ROT Management
This tool-enabled approach fundamentally transforms both sides of the ROT data equation—making debris elimination simultaneously more achievable and more essential.
Why Tool-Enabled AI Makes ROT Management Easier
API Access to Scattered Information
Traditional ROT management failed partly because information remained siloed across dozens of disconnected systems. Modern AI with API access through protocols like MCP can coordinate across these boundaries, creating comprehensive governance that matches the scattered nature of enterprise content.
When a document exists in seventeen locations, tool-enabled AI doesn't just identify this redundancy—it can retrieve all versions, determine the authoritative copy, consolidate necessary metadata, and eliminate unnecessary duplicates through direct interaction with repository APIs.
Workflow Orchestration and Task Delegation
Information governance involves complex, multi-step processes that previously required extensive human coordination. Agentic systems excel at orchestrating these workflows—delegating specialized tasks to appropriate tools, integrating results, and ensuring complete process execution.
For obsolete content, this might involve checking update status against authoritative sources, identifying all dependent documents, generating appropriate notifications, handling exceptions requiring human intervention, and executing disposition actions for eligible content.
Scalable Execution with Consistent Application
The greatest limitation of human-centered governance was scale—organizations simply couldn't hire enough qualified personnel to manually review billions of content items. Tool-enabled AI eliminates this constraint by applying consistent governance at virtually unlimited scale through automated execution.
Rather than sampling small content subsets or focusing only on high-risk repositories, tool-enabled systems can continuously process entire information ecosystems with unwavering policy adherence.
Why Tool Integration Makes Addressing ROT More Critical
The Training Data Quality Imperative
While tool-enabled AI makes ROT management easier, it simultaneously makes addressing digital debris more urgent. AI systems learn from the data they encounter. When these systems integrate with enterprise repositories filled with redundant, obsolete, or trivial information, they absorb and potentially amplify these deficiencies.
Organizations planning AI transformations must recognize that ROT elimination isn't merely about storage savings—it's about ensuring AI systems have high-quality information from which to learn. Tool-enabled systems trained on clean, accurate, relevant content deliver exponentially better results than those contaminated with digital debris.
Recursive Improvement Potential
Tool-enabled AI creates opportunities for recursive improvement impossible with standalone language models. As these systems interact with enterprise content through tools, they generate high-quality execution data that further improves their performance.
This creates a virtuous cycle where better governance leads to cleaner repositories, which enable more effective AI, which further enhances governance capabilities. Conversely, organizations that fail to address ROT data risk a negative spiral where contaminated systems propagate and amplify existing information deficiencies.
Risk Amplification Through Tool Access
When AI systems gain the ability to use tools, the liability associated with ROT data increases dramatically. A standalone language model might identify sensitive information in inappropriate locations, but a tool-enabled system could potentially take actions based on this information—amplifying the risk profile of uncontrolled content.
This dynamic makes proactive governance essential rather than optional for organizations deploying tool-enabled AI systems.
Implementing Tool-Enabled Governance in Large Organizations
Government agencies and large enterprises face distinct challenges when implementing tool-enabled information governance. These organizations typically have the most severe ROT data problems due to their scale, complexity, and longevity. They also often operate under the strictest regulatory frameworks and security requirements.
The following phased approach addresses these unique considerations:
1. Tool Discovery and Integration Mapping
Begin by cataloging existing information management tools and developing an integration strategy through MCP or similar protocols. Most large organizations already possess specialized capabilities for classification, records management, and content analysis—the key is making these accessible to AI systems rather than replacing them.
This approach leverages existing investments while creating a foundation for coordinated governance. When implemented effectively, the whole becomes greater than the sum of its parts as previously isolated capabilities become elements of comprehensive workflows.
2. Small-Scale Pilot with High-Value Targets
Rather than attempting enterprise-wide transformation immediately, identify specific high-value repositories where tool-enabled governance can demonstrate clear ROT reduction benefits. Common candidates include:
- Legal hold repositories with substantial obsolete content
- Project directories with significant duplication
- Departmental drives with minimal existing governance
- Legacy systems scheduled for decommissioning
Focus initially on scenarios where tool-enabled systems can produce measurable improvements with minimal risk. This builds organizational confidence while refining integration approaches.
3. Workflow Composition and Governance Rules
Tool-enabled systems require explicit governance rules that formalize previously implicit organizational knowledge. This process often reveals policy contradictions or gaps previously hidden in manual processes.
When defining these rules, focus on encoding organizational wisdom rather than mere rule compliance. The most effective governance frameworks capture not just regulatory requirements but institutional knowledge about content value, contextual dependencies, and risk considerations specific to your organization.
4. Supervised Automation with Escalation Paths
As tool-enabled systems expand across the enterprise, implement supervised automation with clear escalation paths for exceptional scenarios. Define explicit boundaries between decisions these systems can make autonomously versus those requiring human oversight.
This approach balances efficiency with appropriate controls, particularly important for government agencies and regulated industries where governance decisions may have legal implications. The goal is augmenting human expertise rather than replacing it entirely.
The Future of Tool-Enabled Information Governance
Organizations that successfully implement tool-enabled governance will discover benefits beyond mere ROT reduction. These systems create foundations for broader enterprise intelligence that transforms information from liability into strategic asset.
Knowledge workers gain productivity through reliable, consistent access to authoritative content. Compliance teams reduce risk through comprehensive visibility and evidence-based disposition. IT departments optimize infrastructure by eliminating redundancies and consolidating storage.
Perhaps most importantly, these organizations position themselves for subsequent AI transformations that remain impossible amid digital debris. Clean, well-governed information enables knowledge discovery, process automation, and decision support capabilities that create sustained competitive or operational advantages.
The technology exists today through the convergence of language models, agentic systems, and tool integration protocols. The question is whether your organization will leverage these capabilities before drowning in its own digital debris.
After all, even the most advanced AI needs the right tools to transform intelligence into action—just like we do.
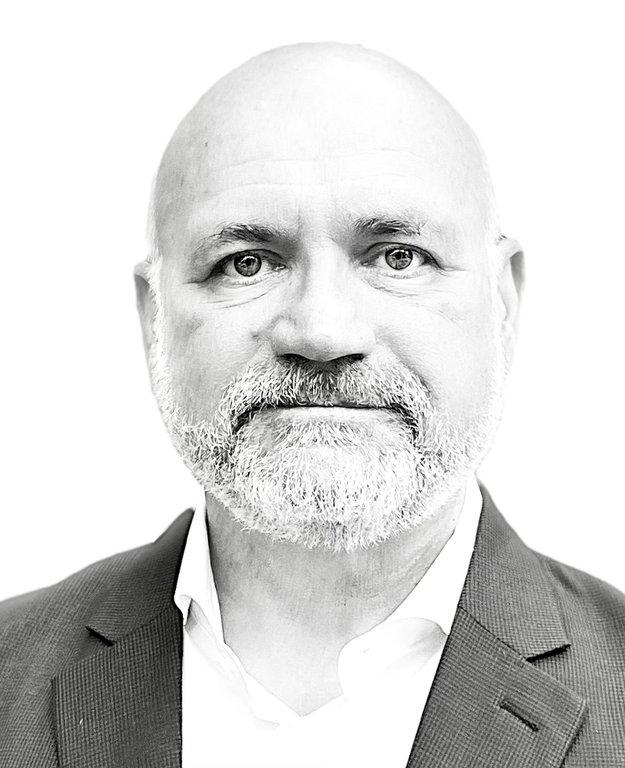
Geordie
Known simply as Geordie (or George, depending on when your paths crossed)—a mononym meaning "man of the earth"—he brings three decades of experience implementing enterprise knowledge systems for organizations from Coca-Cola to the United Nations. His expertise in semantic search and machine learning has evolved alongside computing itself, from command-line interfaces to conversational AI. As founder of Applied Relevance, he helps organizations navigate the increasingly blurred boundary between human and machine cognition, writing to clarify his own thinking and, perhaps, yours as well.
No comments yet. Login to start a new discussion Start a new discussion